Kurser inom databaser och analys
Stärk dina kunskaper inom databaser och Business Intelligence. Våra kurser täcker SQL Server, Power BI och Azure för administratörer, utvecklare och BI-specialister. Lär dig allt från Transact-SQL till Data Science, prestandaoptimering och moderna dataplattformar som Azure och AWS.
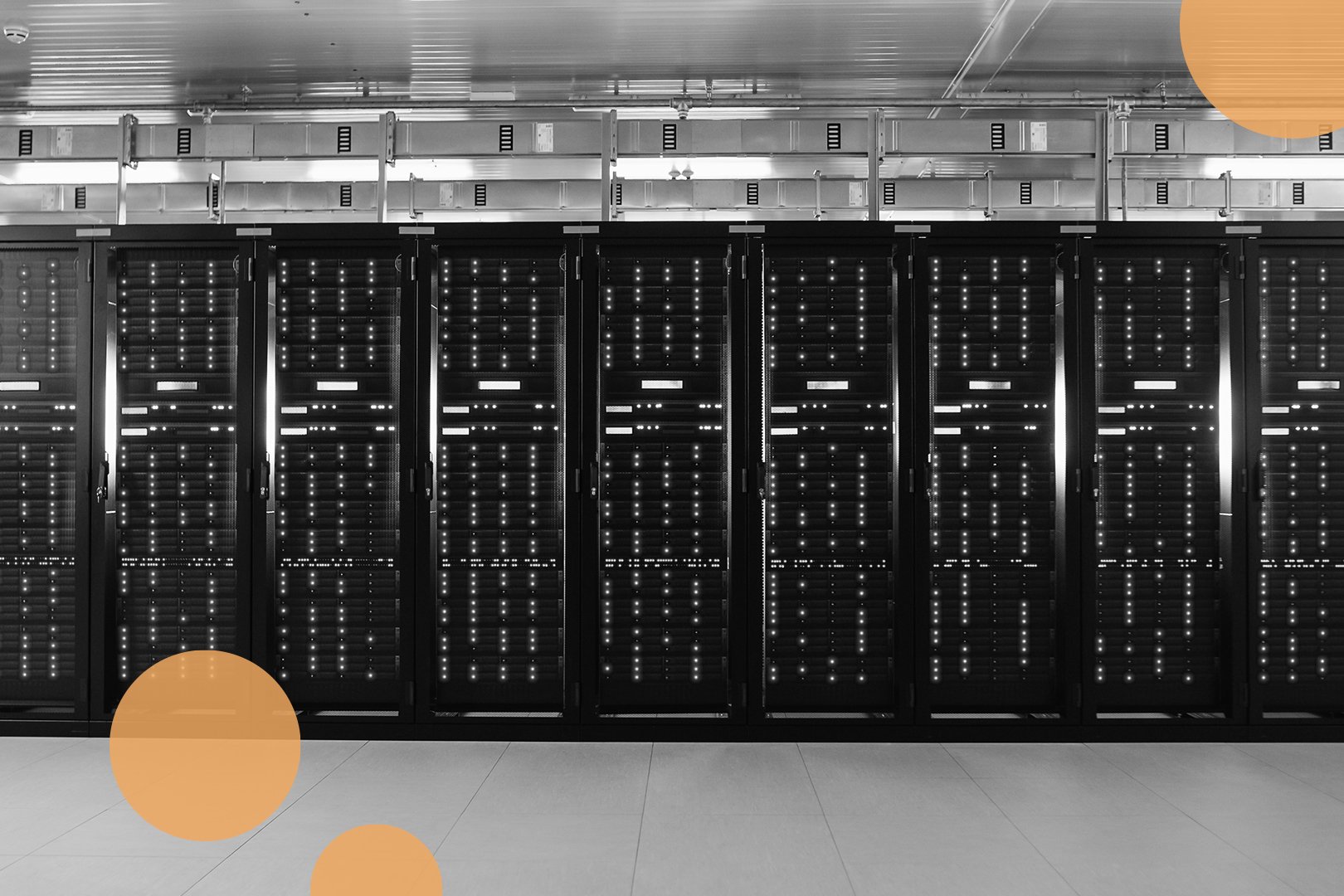
Utbildningar inom databaser och Business Intelligence
Vill du ta din kompetens inom databaser och BI till nästa nivå? Våra utbildningar är designade för både nybörjare och erfarna yrkesverksamma som arbetar med databasadministration, utveckling eller analys.
Vi erbjuder ackrediterad Microsoft-utbildning inom SQL Server, Power BI och Azure som dataplattform för SQL-administratörer, SQL-utvecklare och BI-specialister. Våra kurser täcker viktiga ämnen som:
- Transact-SQL och SQL-administration
- Backup/restore och prestandaoptimering
- Data Science och Data Engineering
- Azure Data Factory, Cosmos DB och Microsoft Fabric
Utöver Microsoft hittar du även kurser inom dataområdet från AWS och Oracle, vilket gör det enkelt för dig att välja den kompetens som bäst matchar din roll och dina mål.